Image Sequence Recognition
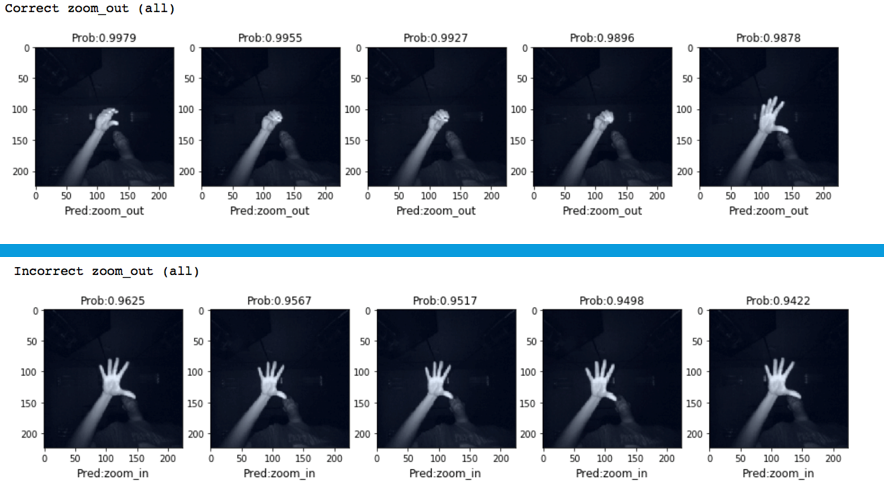
Abstract
​
Achieving high accuracy is an important objective in image sequence classification applications. This project explores four approaches to achieve high accuracy in image sequence recognition:
​
1) Using pre-trained models like RESNET18 on image sequences.
2) Explore normalised timestamp regression to first tag the image in a sequence to its relative position in the sequence and then use this number to classify the image to a sequence label.
3) Use 2D-CNN to extract features from the images and LSTM to analyse the sequential nature of the images.
4) Overlay images in one sequence to capture the temporal features as 2-dimensional spatial ones and then build a 2D-CNN model on these overlaid images.
​
These approaches are evaluated on the overall accuracy, F1 score and confusion matrix for 11 gesture classes. It is observed that CNN-LSTM model has the best overall and inter-class accuracy.
​
Credits:
With collaboration with Apoorva Joshi, Chi Cheng, Deepika Jaswal and Jyoti Bukkapatil.
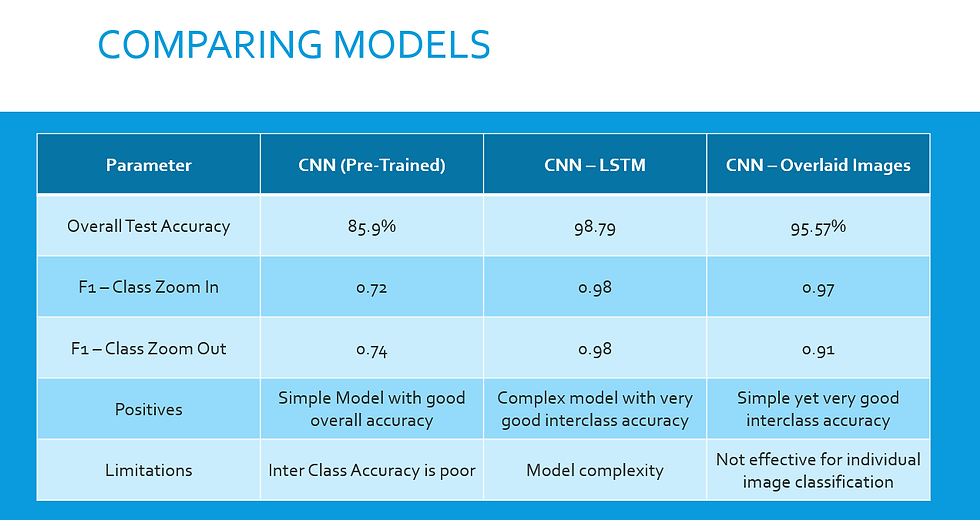